بوابة مؤتمرات الجامعة
حــــــول الجـــــامعة
تاأسست جامعة القادسية عام 1987 بكليتين هما كلية التربية وكلية الإدارة والاقتصاد وفي العام الدراسي الثاني أُفتتحت كلية الآداب. وسرعان ما أُفتتحت كليات اخرى في مركز محافظة القادسية وكذلك مركز محافظتي واسط والمثنى ومن كلياتها هذه ماأصبح نواة جامعة مستقلة هي جامعة واسط. ولاحقا جامعة أخرى في السماوة باسم جامعة المثنى.وعلى مدى سنوات عمرها ازدادت كلياتها وتمت أقسامها وتطورت مختبراتها وتضم كلياتها اليوم…المزيد
رؤية ورسالة واهداف جامعة القادسية
بسم الله الرحمن الرحيم
الحمد لله رب العالمين والصلاة والسلام على أشرف الخلق والمرسلين محمد وآله الطاهرين
بآسم الهيأة التعليمية والإدارية بجامعة القادسية أبارك لطلبتنا الميامين ، تخرجهم للعام المنصرم ، لنستقبل العام الجديد بعودة ميمونة الى الدوام الحضوري ، الذي يعيد للمشهد الاكاديمي رونقه الواقعي وفاعليته بالمشاركة الجادة لصناعة مستقبل زاخر بالمعرفة والمجد. ففي الوقت الذي كللت فيه جامعتنا الغراء مسيرتها السنوية بذكراها الخامسة والثلاثين لتأسيس صرحها العلمي الرصين بالعام الماضي … فإننا نود التذكير بأن هذا الصرح الكبير لم يكن يبلغ هذه المكانة السامقة لولا جهود الأساتذة الرواد ، الأحياء منهم ، والراحلين الى جوار ربهم ، قياداتً وأساتذةً ومنتسبين.
“مؤسسات التعليم العالي والبحث العلمي في العراق لم يعد طموحها وبرامجها الأكاديمية مقتصرا على البيئة المحلية فحسب بل إن مؤشرات تفاعلها العالمي لم يعد خافيا على المراقبين والمتابعين الذين يقرأون مستوى الأداء ومعطيات النشر العالمي ونتائج التصنيفات الدولية ولاسيما إشادة اليونسكو بالتحول المهم على صعيد الأنظمة التعليمية في الجامعات العراقية فضلا عن التميز في مجال التعاون الوثيق مع برامج الأمم المتحدة في مجال المشاريع المشتركة ذات الأثر المباشر في حقل التدريب وتطوير المهارات.”
احصائيات الجامعة
الالقاب العلمية
الهيئة التدريسية
الدراسات العليا
الدراسة الاولية
ادرس في العراق
مركز الحاسبـــــة
مستودع البحوث
مسار بولونيا
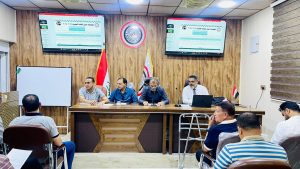
مدير قسم النشاطات الطلابية بجامعة القادسية يكلف بمهام ادارة المؤتمر الفني لدوري الفئات العمرية 2024-2025 .
26/06/2025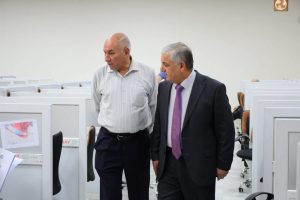
رئيس جامعة القادسية يتفقد سير الامتحانات النهائية لطلبة المرحلة السادسة في كلية الطب
26/06/2025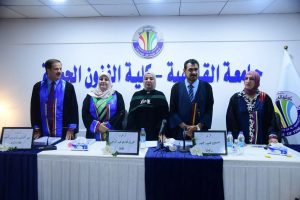
رسالة ماجستير في كلية الفنون الجميلة بجامعة القادسية ناقشت فاعلية استراتيجية خلايا التعلم في التفكير الناقد
26/06/2025موقعنا على الخارطة
مجلاتنا العلمية
- مجلة القادسية لعلوم الطب البيطريمجلة علمية نصف سنوية تصدر عن كلية الطب البيطري
- مجلة القادسية لعلوم الحاسوب والرياضياتمجلة علمية نصف سنوية تصدر عن كلية علوم الحاسوب وتكنلوجيا المعلومات
- مجلة القادسية الطبيةمجلة علمية نصف سنوية تصدر عن كلية الطب
- مجلة القادسية للعلوم الانسانيةمجلة علمية نصف سنوية تصدر عن كلية الاداب
- مجلة القادسية للعلوم الزراعيةمجلة علمية نصف سنوية تصدر عن كلية الزراعة